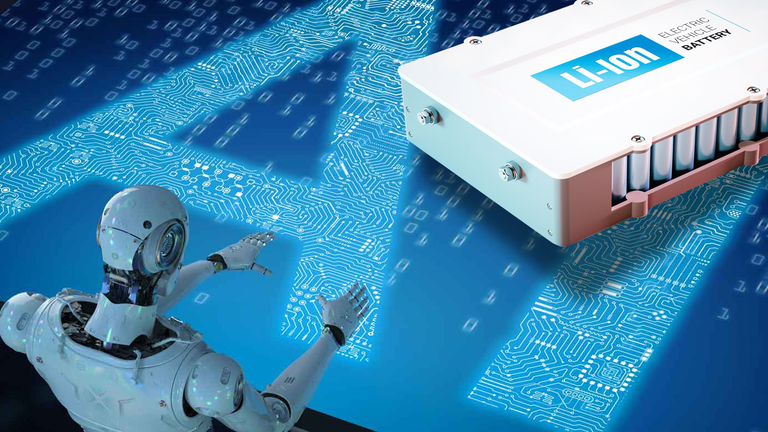
12 Mar Machine Learning Helps In Interpreting Battery Life
A study carried out jointly by Stanford University, SLAC National Accelerator Laboratory, the Massachusetts Institute of Technology, and the Toyota Research Institute (TRI) demonstrated the use of machine learning algorithms to understand the lifecycle of lithium-ion batteries. Until now, machine learning in battery technology was limited to identifying patterns in data to speed up scientific analysis. The latest discovery will help researchers in designing and developing longer-lasting batteries.
ML And Battery Life Cycle
The research team has been working to develop a long-lasting electric vehicle battery that can be charged in 10 minutes.
“Battery technology is important for any type of electric powertrain. By understanding the fundamental reactions that occur within the battery we can extend its life, enable faster charging and ultimately design better battery materials. We look forward to building on this work through future experiments to achieve lower-cost, better-performing batteries,” said Patrick Herring, a senior scientist of Toyota Research Institute.
Earlier studies used more conventional machine learning forms to accelerate battery testing and find out the best charging method. Though the studies made major progress in determining battery lifetime, they did not reveal much about the science behind why a few batteries last longer than the others.
The current research teaches machines how to learn a new type of failure mechanism to design better and safer fast-charging batteries. In general, fast charging stresses and damages the battery. Better practices would help battery technology and fight climate change, the team said. Further, this approach can be used for developing grid-scale battery systems for producing wind and solar electricity.
The team was able to optimize the fast charging protocol for lithium-ion batteries within a month using machine learning. Without ML, this would usually take two years. “At the end of the day, we see our job as accelerating the pace of battery R&D. Whether it’s discovering new chemistry or finding a way to make a safer battery, it’s all very time-consuming. We’re trying to save time,” said Will Chueh, an associate professor at Stanford University, who also led the study.
The Experiment
For this experiment, the team took a closer look at the Lithium ions movement between the cathode and anode — made of nano-sized grains lumped together as particles — during charging and discharging. In particular, the behavior of cathode particles, comprising nickel, manganese, and cobalt (NMC), were observed in detail. NMC is the most widely used material in electric vehicle batteries. The particles absorb lithium ions when the battery is discharging and release them when the battery is charging.
The team got an overall look at the particles when the battery was being fast charged using X-rays from SLAC’s Stanford Synchrotron Radiation Lightsource. The same particles were later examined with scanning X-ray transmission microscopy, which focuses on individual particles. The data obtained from these experiments; information from mathematical models on fast charging; and physics and chemistry equations were used in the scientific machine learning algorithm. The team said this is the first time scientific machine learning has been used in battery technology.